The team has been working on the application of artificial intelligence in the future automotive field for more than a decade. The research focuses on three key areas: multi-objective control, human-machine interaction, and cyber-physical systems, all of which are aimed at achieving net zero emissions and trustworthy engineering solutions. The overall goal is to promote the development of future cars that are not only zero-emission, but also trustworthy and skilfully utilize digitalization at all operational levels.
The research aims to address challenges at four observation scales to pave the way for innovative solutions in the dynamic landscape of automotive technology:
- power unit level: engine/battery/fuel cell (physical/data-enhanced robust modelling)
- Powertrain level: series/parallel/power split hybrid (learning-based energy management and module design)
- Human-Vehicle level: driver/vehicle/road (driving behaviour recognition and human-machine interaction control)
- Traffic level: fleet and traffic system (following vehicle characterization, fleet control and traffic system allocation, etc)
The selected key findings show the various research in the four levels of the advanced vehicle and transportation development. The details of the specific research in each level are introduced step by step.
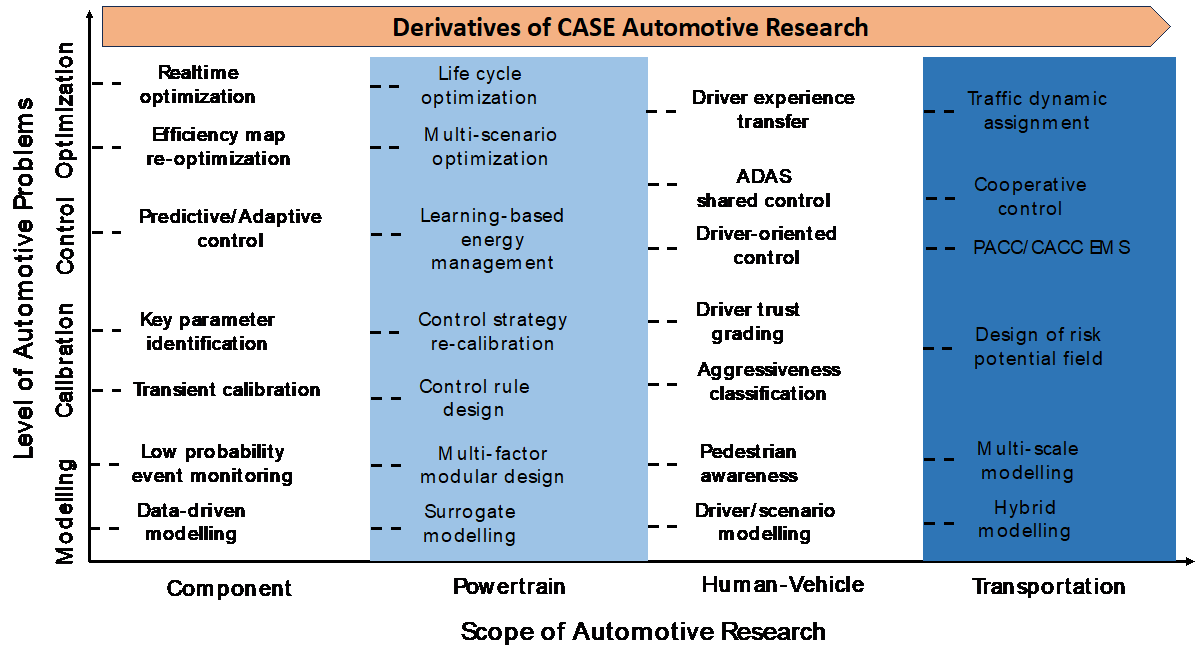
1. Component level
(a) Component Modelling
Among the relevant research of our research centre in the powertrain component modelling, the artificial intelligent technology and digital methods are applied widely in various new-energy vehicles. The three main aspects are the dedicated hybrid engine, the vehicle battery and the vehicle fuel cell. The selected key findings of component modelling are shown as: 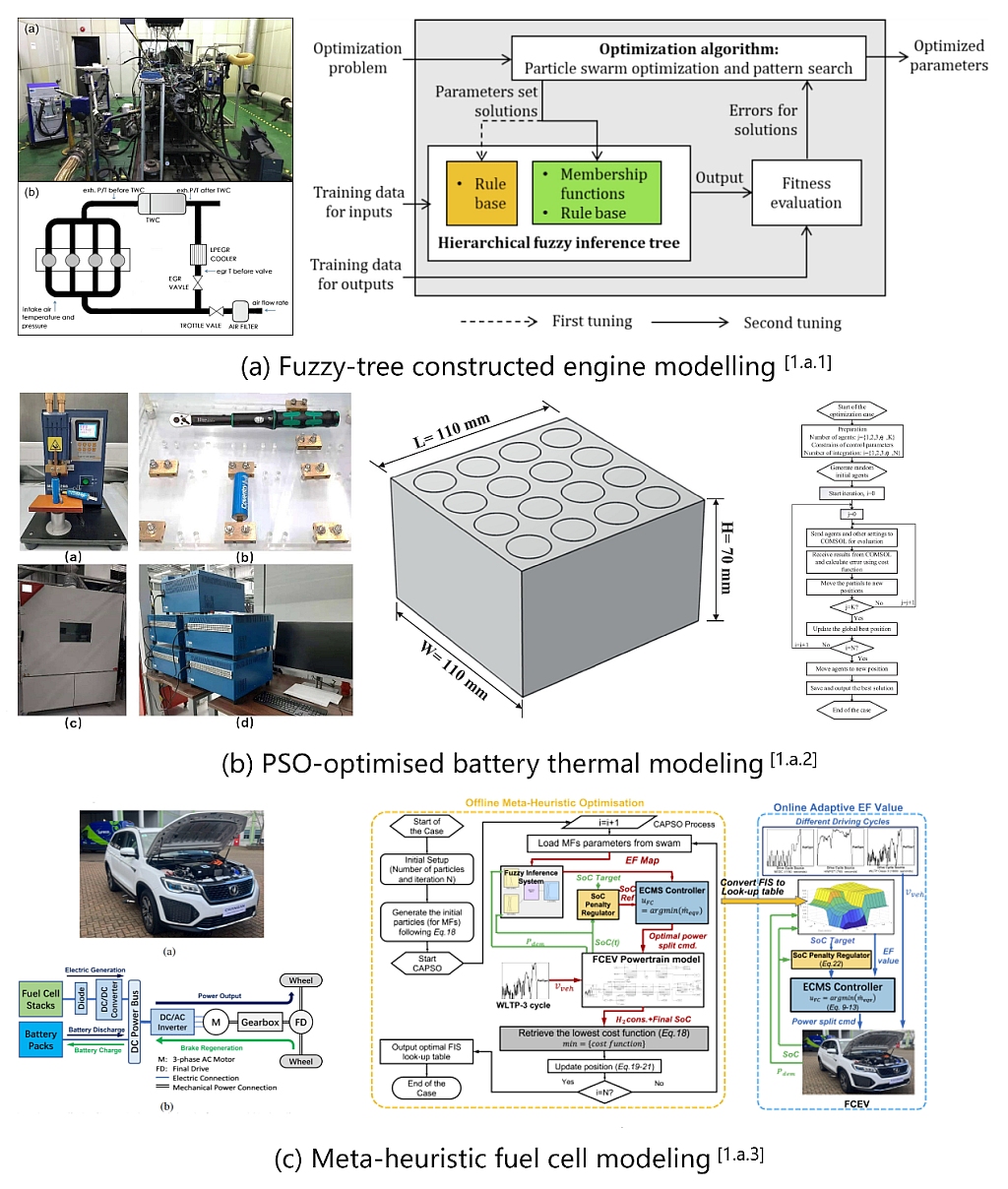
References
[1.a.1] He, X., et.al. (2023). Robust key parameter identification of dedicated hybrid engine performance indicators via K-fold filter collaborated feature selection. Engineering Applications of Artificial Intelligence, 126, 107114.
[1.a.2] Sun, Z., et.al. (2023). Algorithm-driven optimization of lithium-ion battery thermal modeling. Journal of Energy Storage, 65, 107388.
[1.a.3] Zhang, F., et.al. (2024). Meta-heuristic Adaptive Equivalent Consumption Minimization of a Fuel Cell Vehicle Incorporating Fuzzy Inference and Particle Swarm Optimization. IEEE Transactions on Transportation Electrification.
(b) Component Control
The research work of the centre is mainly based on the transient control of the engine, focusing on the efficient and robust transient control of powertrain components. The typical findings are selected as follows:
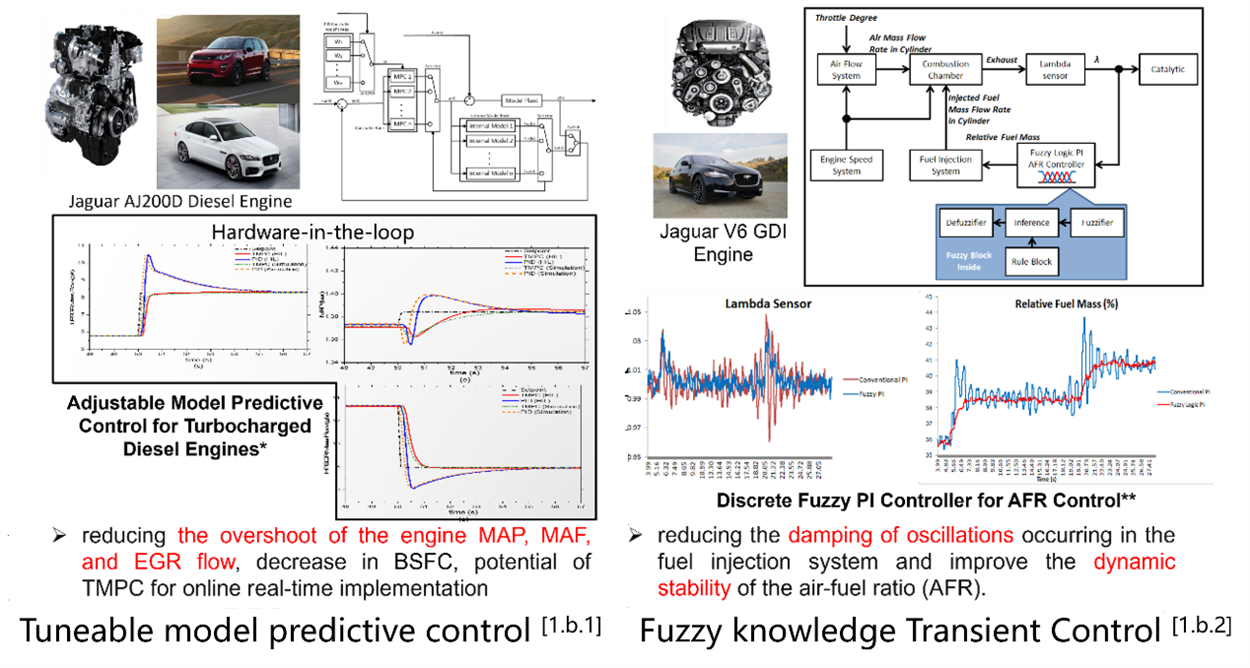
References
[1.b.1] Zhang, Y., et.al. (2018). Tuneable model predictive control of a turbocharged diesel engine with dual loop exhaust gas recirculation. Proceedings of the Institution of Mechanical Engineers, Part D: Journal of Automobile Engineering, 232(8), 1105-1120.
[1.b.2] Li, Z., et.al. (2019). Intelligent air/fuel ratio control strategy with a PI-like fuzzy knowledge–based controller for gasoline direct injection engines. Proceedings of the Institution of Mechanical Engineers, Part D: Journal of Automobile Engineering, 233(8), 2161-2173.
(c) Component Monitoring
The research work of the centre is mainly based on the component monitoring via computer vision algorithm, focusing on the efficient and robust artificial intelligent method to monitor the working status of the components and detect the diagnosis. The typical findings are selected as follows:
Deep learning-driven analysis for cellular structure characteristics of spherical premixed hydrogen-air flames [1.c.1] [1.c.2]
References
[1.c.1] Zhang, G., Xu, H., Wu, D., Yang, J., Morsy, M. E., Jangi, M., ... & Kim, W. (2024). Deep learning-driven analysis for cellular structure characteristics of spherical premixed hydrogen-air flames. International Journal of Hydrogen Energy, 68, 63-73.
[1.c.2] Zhang, G., Xu, H., Wu, D., Yang, J., Morsy, M. E., Jangi, M., & Cracknell, R. (2024). Quantitative three-dimensional reconstruction of cellular flame area for spherical hydrogen-air flames. Fuel, 375, 132504.
2. Powertrain level
(a) Component Sizing
The research work of the centre mainly focuses on the optimal selection of powertrain components for different hybrid models, using a variety of advanced optimization algorithms and applying them to the combination design of different types of components. The selected typical findings are shown below:
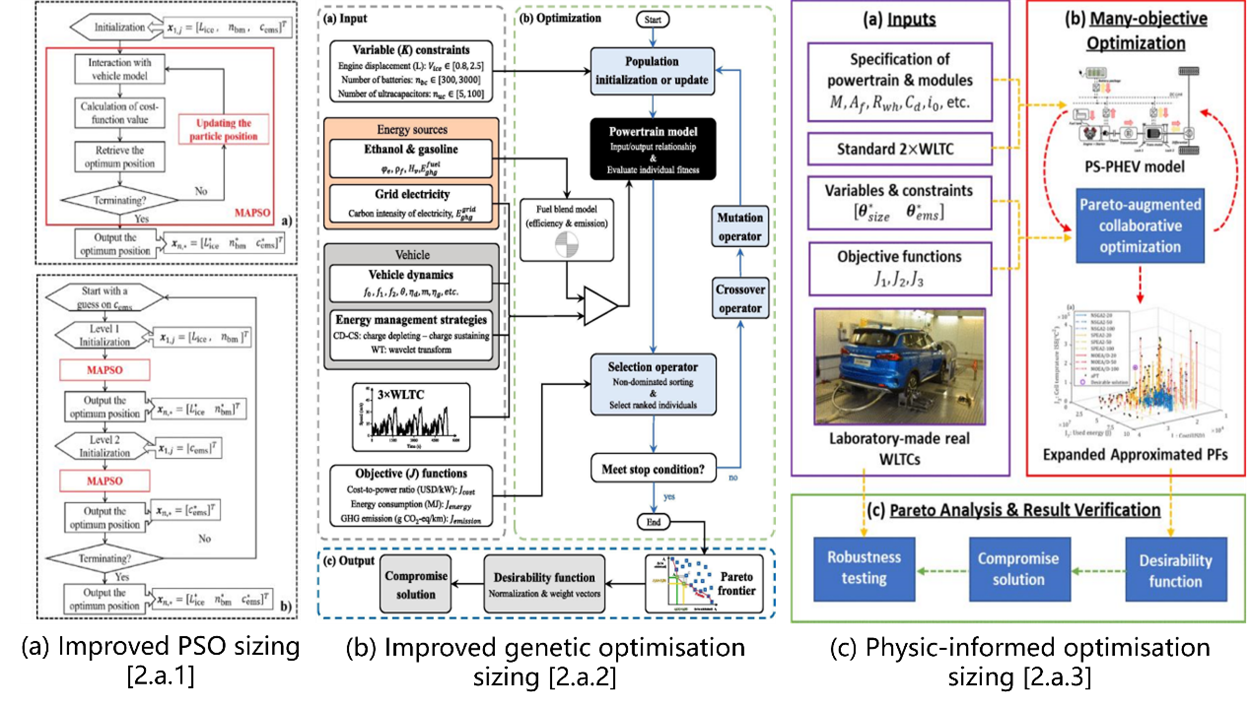
References
[2.a.1] Zhou, Q., et.al. (2020). Modified particle swarm optimization with chaotic attraction strategy for modular design of hybrid powertrains. IEEE transactions on transportation electrification, 7(2), 616-625.
[2.a.2] He, Y., et.al. (2020). Multiobjective component sizing of a hybrid ethanol-electric vehicle propulsion system. Applied energy, 266, 114843.
[2.a.3] Li, J., et.al. (2022). Electrothermal dynamics-conscious many-objective modular design for power-split plug-in hybrid electric vehicles. IEEE/ASME Transactions on Mechatronics, 27(6), 4406-4416.
(b) Power Management System
Multiple machine learning methods are applied to achieve the robustness-enhanced and generic EMS for different hybrid electric vehicles efficiently. The selected typical findings are shown below:

References
[2.b.1] Zhou, Q., et.al. (2022). Transferable representation modelling for real-time energy management of the plug-in hybrid vehicle based on k-fold fuzzy learning and Gaussian process regression. Applied energy, 305, 117853.
[2.b.2] Li, J., et.al. Cyber-physical data fusion in surrogate-assisted strength pareto evolutionary algorithm for PHEV energy management optimization. IEEE Transactions on Industrial Informatics, 18(6), 4107-4117.
[2.b.3] Shuai, B., et.al. (2020). Heuristic action execution for energy efficient charge-sustaining control of connected hybrid vehicles with model-free double Q-learning. Applied energy, 267, 114900.
3. Human-Vehicle level
(a) Driver-oriented Powertrain Control
Focusing on the driver-oriented technology, the various research is developed, including the style/pattern identification, predictive control and adaptive control. The selected relevant findings are shown as follows:
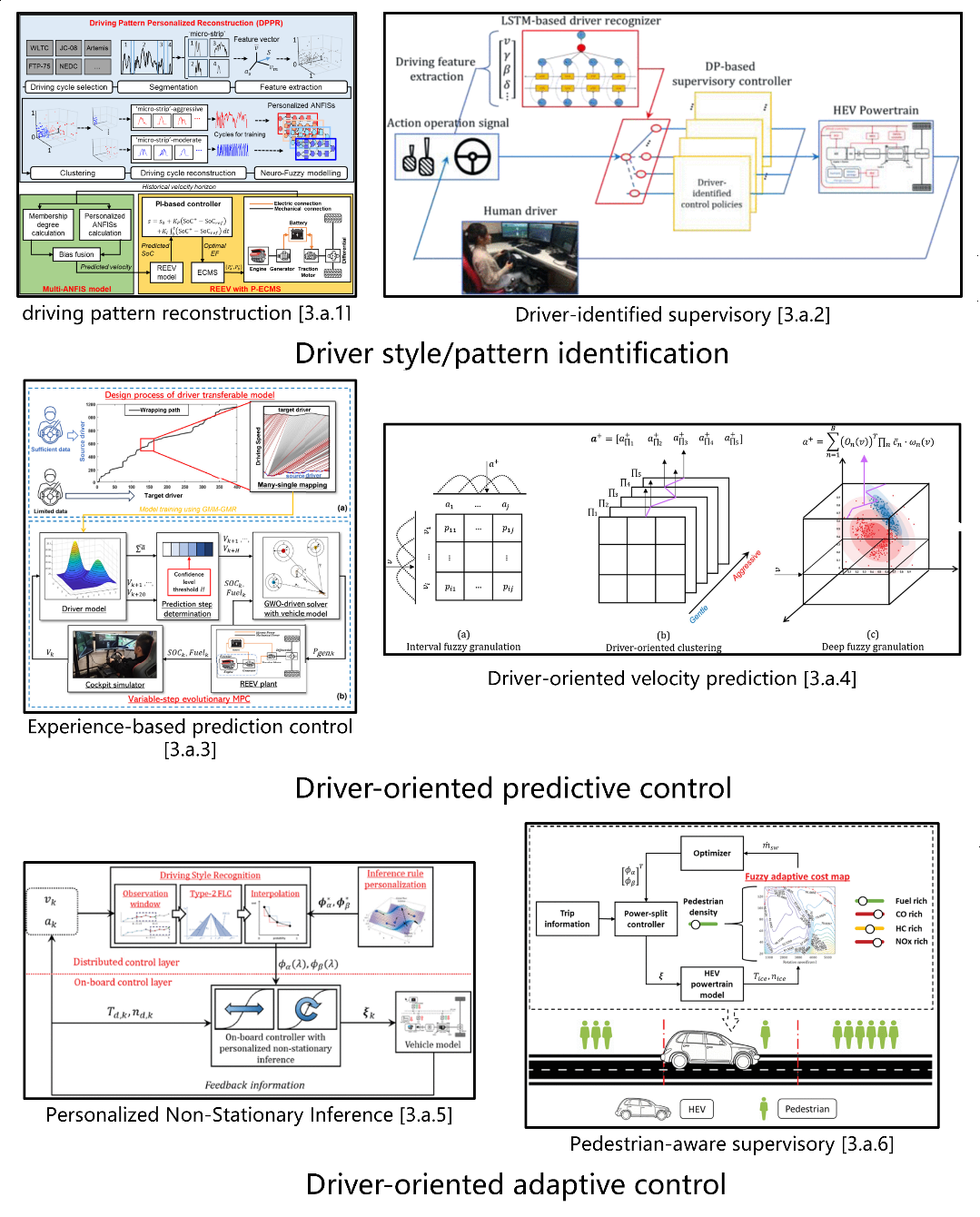
References
[3.a.1] Zhang, Y., et.al. (2024). Predictive equivalent consumption minimization strategy based on driving pattern personalized reconstruction. Applied Energy, 367, 123424.
[3.a.2] Li, J., et.al. (2020). Driver-identified supervisory control system of hybrid electric vehicles based on spectrum-guided fuzzy feature extraction. IEEE Transactions on Fuzzy Systems, 28(11), 2691-2701.
[3.a.3] Li, J., et.al. (2024). Experience-Shared Variable-Step Predictive Control of Range-Extended Electric Vehicles Using Transferable Driver Model. IEEE Transactions on Intelligent Transportation Systems.
[3.a.4] Li, J., et.al. (2019). Dual-loop online intelligent programming for driver-oriented predict energy management of plug-in hybrid electric vehicles. Applied Energy, 253, 113617.
[3.a.5] Li, J., et.al. (2021). Distributed cooperative energy management system of connected hybrid electric vehicles with personalized non-stationary inference. IEEE transactions on transportation electrification, 8(2), 2996-3007.
[3.a.6] Li, J., et.al. (2021). Pedestrian-aware supervisory control system interactive optimization of connected hybrid electric vehicles via fuzzy adaptive cost map and bees algorithm. IEEE Transactions on Transportation Electrification, 8(2), 2959-2970.
(b) Vehicle-Driver Shared Control
By applying the driver-in-the-loop platform, the various human-machine shared control methods or other advanced driver assistance systems (ADASs) are developed for safer, more comfort and fuel-efficient driving.
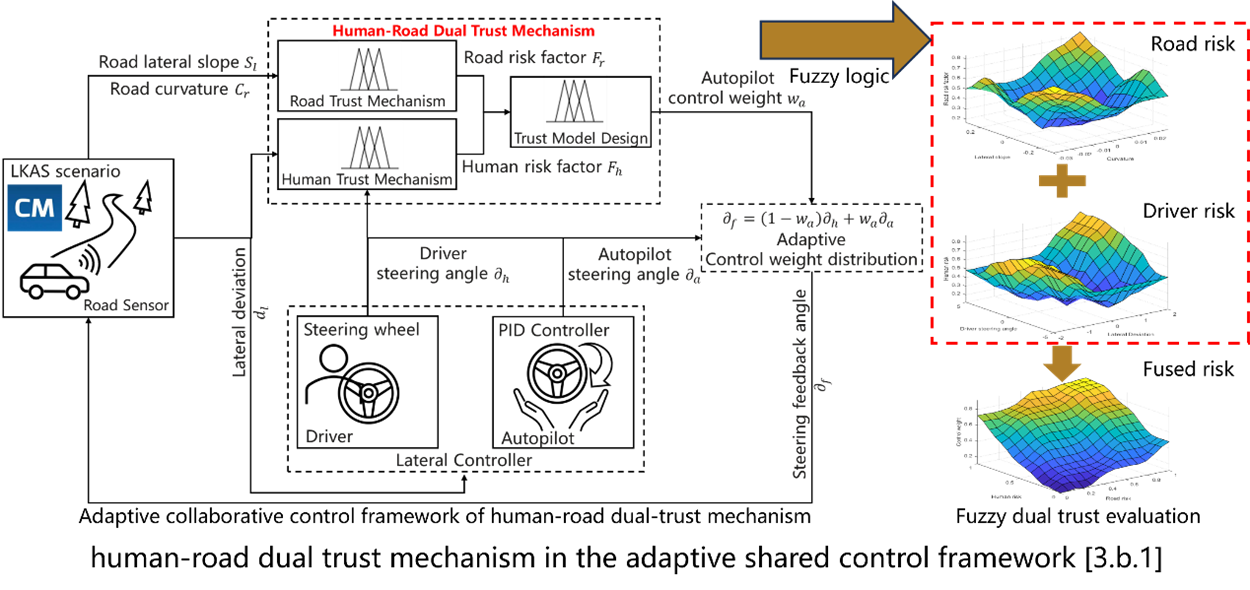
References
[3.b.1] He, X., et.al. (2023). Human-Road Dual Trust Mechanism in Adaptive Distributed Shared Control Framework under Lane Keeping Scenario. In 2023 7th CAA International Conference on Vehicular Control and Intelligence (CVCI) (pp. 1-6). IEEE.
4. Fleet level
(a) EMS with CACC
In advanced driver assistance systems, the combination of energy management system (EMS) and collaborative adaptive cruise control (CACC) is an important research area. The relevant research direction of this research centre is to use AI technology to integrate and analyse real-time data from multiple sources to provide decision support for EMS, optimize energy consumption and improve overall vehicle performance. This integration of technology not only improves driving safety, but also effectively reduces energy consumption and emissions, and promotes the development of intelligent transportation systems. The selected findings are shown below:
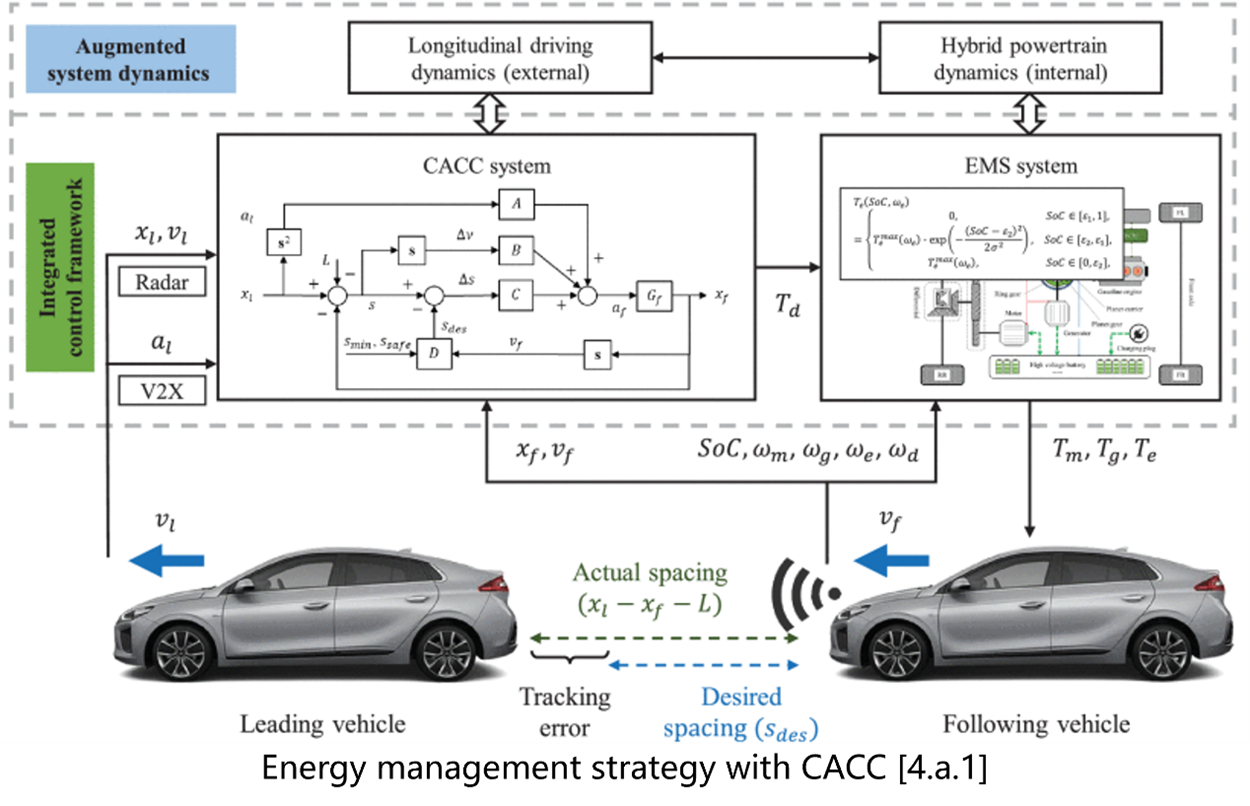
References
[4.a.1] He, Y., et.al. (2020). Multiobjective co-optimization of cooperative adaptive cruise control and energy management strategy for PHEVs. IEEE Transactions on Transportation Electrification, 6(1), 346-355.
(b) Multi-objective Control with Platoon
Considering the different factors of the platoon, including the individual vehicle status, the driver specification and others, the various multiobjective predictive control methods are developed to keep the optimal trade-off among fuel economy, comfort and safety.
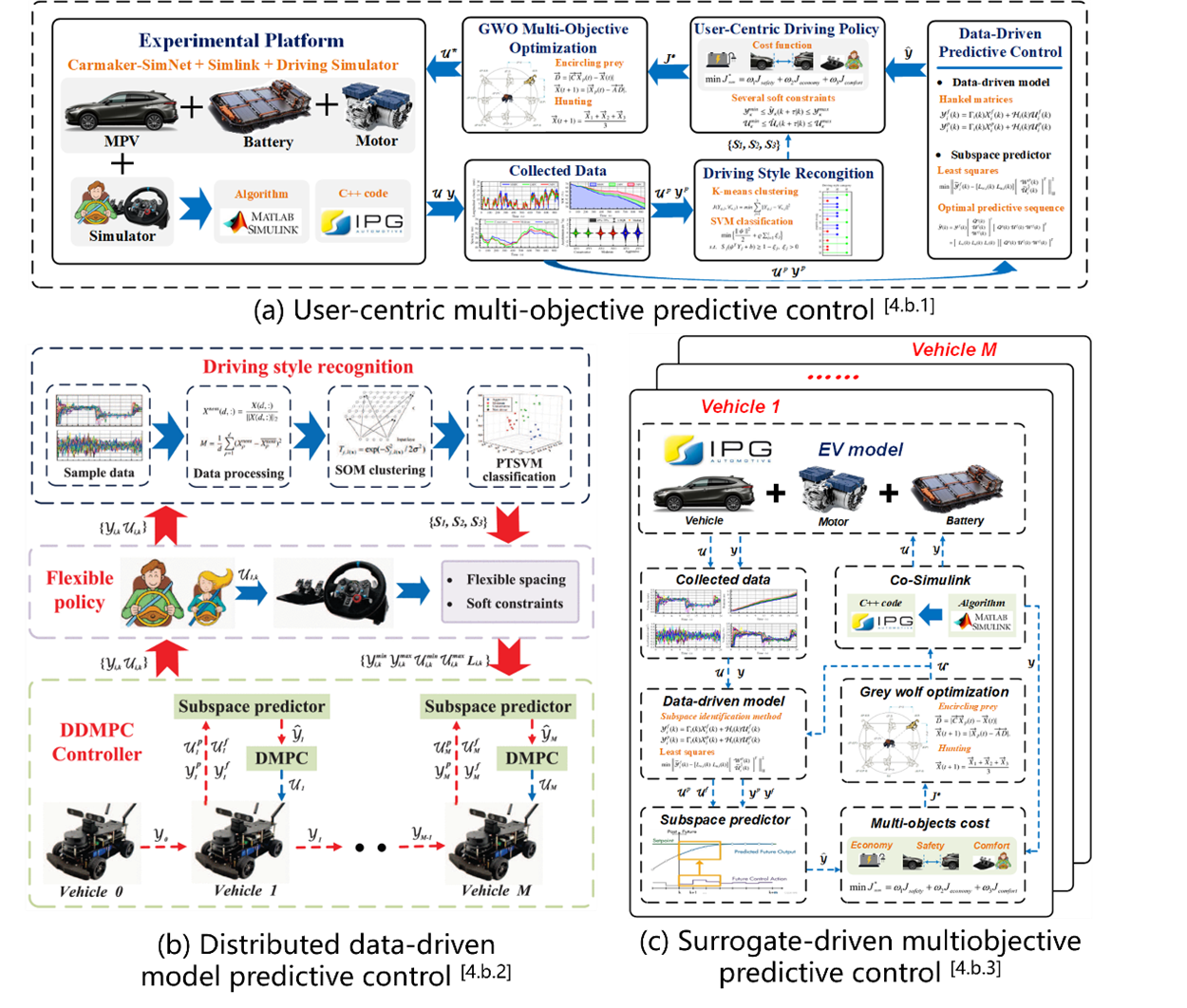
References
[4.b.1] Wu, Y., et.al. (2024). User-Centric Multi-Objective Predictive Control for Mixed Vehicular Platoon. IEEE Transactions on Intelligent Vehicles.
[4.b.2] Wu, Y., et.al. (2024). Driver-Centric Data-Driven Model Predictive Vehicular Platoon With Longitudinal-Lateral Dynamics. IEEE Transactions on Intelligent Transportation Systems.
[4.b.3] Wu, Y., et.al. (2024). Surrogate-Driven Multi-Objective Predictive Control for Electric Vehicular Platoon. IEEE Transactions on Transportation Electrification.